
Ren, Hui, Joanna Materzynska, Rohit Gandikota, David Bau, and Antonio Torralba. 2024. “Art-Free Generative Models: Art Creation Without Graphic Art Knowledge.” http://arxiv.org/abs/2412.00176.
アートを含まない学習データを学習したAIモデルをベースに、少数のアート作品の画像でLoRAを学習。きちんとそのアーティストの特徴を掴んだ画像が生成された。
2024

Caillon, Antoine, and Philippe Esling. 2021. “RAVE: A Variational Autoencoder for Fast and High-Quality Neural Audio Synthesis.” arXiv [cs.LG]. arXiv. http://arxiv.org/abs/2111.05011.
2019

Hicks, Michael Townsen, James Humphries, and Joe Slater. 2024. “ChatGPT Is Bullshit.” Ethics and Information Technology 26 (2). https://doi.org/10.1007/s10676-024-09775-5.
ChatGPTの本質を、哲学者のフランクファートが提唱した概念、「ブルシット=ウンコな議論」を通して捉え直す
2024

Liu, Xubo, Zhongkai Zhu, Haohe Liu, Yi Yuan, Meng Cui, Qiushi Huang, Jinhua Liang, et al. 2023. “WavJourney: Compositional Audio Creation with Large Language Models.” arXiv [cs.SD]. arXiv. http://arxiv.org/abs/2307.14335.
LLMと複数の音声合成モデルを駆使して、テキストプロンプトからスピーチ、音楽、SEなどを含む音のコンテンツ(ラジオドラマ、ポッドキャストのようなもの)を生成
2023

Bralios, Dimitrios, Gordon Wichern, François G. Germain, Zexu Pan, Sameer Khurana, Chiori Hori, and Jonathan Le Roux. 2024. “Generation or Replication: Auscultating Audio Latent Diffusion Models.” In ICASSP 2024 - 2024 IEEE International Conference on Acoustics, Speech and Signal Processing (ICASSP), 1156–60. IEEE.
音楽生成AIは学習データをコピーしているだけではないか? 学習データと生成されたデータを比較。
2024

Broad, Terence, Sebastian Berns, Simon Colton, and Mick Grierson. 2021. “Active Divergence with Generative Deep Learning -- A Survey and Taxonomy.” arXiv [cs.LG]. arXiv. http://arxiv.org/abs/2107.05599.
生成モデル(Generative Models)をはじめとするAIモデルの精度が上がる=学習データのパターンをより忠実に再現、再構成できるようになってきた一方で、創造性の観点から言うとそれって学習データものの焼き直しに過ぎないのでは? とう疑問も。 どう適度な逸脱を図るか… が本論文のテーマ。
2021

Jones, Cameron, and Benjamin Bergen. 2023. “Does GPT-4 Pass the Turing Test?” arXiv [cs.AI]. arXiv. http://arxiv.org/abs/2310.20216.
まだチューリング・テストをクリアしているとは言えなそう… 今、テストを実施する意味とは?
2023

Shan, Shawn, Jenna Cryan, Emily Wenger, Haitao Zheng, Rana Hanocka, and Ben Y. Zhao. 2023. “GLAZE: Protecting Artists from Style Mimicry by Text-to-Image Models.” arXiv [cs.CR]. arXiv. http://arxiv.org/abs/2302.04222.
アーティストのスタイルが勝手に模倣されることを防ぐ Adversarial Example
2023

CLAPを用いることでText-to-AudioのSOTAを達成。オープンソース化されていて、すぐに試せるオンラインデモもあり!
Liu, Haohe, Zehua Chen, Yi Yuan, Xinhao Mei, Xubo Liu, Danilo Mandic, Wenwu Wang, and Mark D. Plumbley. 2023. “AudioLDM: Text-to-Audio Generation with Latent Diffusion Models.” arXiv [cs.SD] . arXiv. http://arxiv.org/abs/2301.12503.
2023

Donahue, Chris, Antoine Caillon, Adam Roberts, Ethan Manilow, Philippe Esling, Andrea Agostinelli, Mauro Verzetti, et al. 2023. “SingSong: Generating Musical Accompaniments from Singing.” arXiv [cs.SD] . arXiv. http://arxiv.org/abs/2301.12662.
音源分離技術を使ってボーカルとそれに付随する伴奏を抽出。その関係を学習。Ground Truth (元々の曲に入ってた伴奏)には流石に劣るがそれに匹敵するクオリティの曲を生成できるようになった。
2023

Schneider, Flavio, Zhijing Jin, and Bernhard Schölkopf. 2023. “Moûsai: Text-to-Music Generation with Long-Context Latent Diffusion.” arXiv [cs.CL] . arXiv. http://arxiv.org/abs/2301.11757.
Latent Diffusionのアーキテクチャを利用して、テキストから音楽を生成するモデル
2023

Agostinelli, Andrea, Timo I. Denk, Zalán Borsos, Jesse Engel, Mauro Verzetti, Antoine Caillon, Qingqing Huang, et al. 2023. “MusicLM: Generating Music From Text.” arXiv [cs.SD] . arXiv. http://arxiv.org/abs/2301.11325.
“a calming violin melody backed by a distorted guitar riff” といったテキストから音楽がサウンドファイルとして生成される. Stable Diffusionの音楽版
2023

Wu, Yusong, Kyle Kastner, Tim Cooijmans, Cheng-Zhi Anna Huang, and Aaron Courville. n.d. “Datasets That Are Not: Evolving Novelty Through Sparsity and Iterated Learning.”
データセットの模倣ではない、新奇性の高い出力を生成AIを用いて実現するために、生成した画像をデータセットに追加していくことを繰り返す
2022

Chen, Gong, Yan Liu, Sheng-Hua Zhong, and Xiang Zhang. 2018. “Musicality-Novelty Generative Adversarial Nets for Algorithmic Composition.” In Proceedings of the 26th ACM International Conference on Multimedia , 1607–15. MM ’18. New York, NY, USA: Association for Computing Machinery.
人真似ではない新しい音楽をAIで生成しようとする野心的な研究
2018

Hayes, B., Saitis, C., & Fazekas, G. (2021). Neural Waveshaping Synthesis.
CPUでもサクサク動くのがポイント!
2021

CLIP-guided collage image optimization using Evolutionary Strategy
画像とテキストがどれくらいマッチしているかを定量化するCLIPモデルを用いて、要素画像の配置を最適化。入力されたテキストにあったコラージュ画像を生成するシステム
2021

Botto Project
CLIP+VQ-GANの仕組みを活用
2021

Bourached, A., & Cann, G. H. (2019). Raiders of the Lost Art. CrossTalk, 22(7–8), 35. https://doi.org/10.1525/9780520914957-028
X線写真をコンテンツ画像に、同時代の同じ作家の絵をスタイル画像としてスタイルトランスファーをかける。美術史家などからその手法に対して強い批判も上がっている。
2019

CLIPからオーディオ表現を抽出する手法であるWav2CLIPを提案。オーディオ分類・検索タスクで良好な結果を残す
2021

YINGTAO TIAN, DAVID HA. Modern Evolution Strategies for Creativity: Fitting Concrete Images and Abstract Concepts (2021)
テキストと画像がどのくらいマッチしているかを定量化するモデル OpenAI CLIPを評価関数に用いて、進化戦略ESによって生成した抽象画
2021

FakeAVCeleb: A Novel Audio-Video Multimodal Deepfake Dataset, Hasam Khalid, Shahroz Tariq, Minha Kim, Simon S. Woo (2021)
顔をディープフェイク技術で動かし、リップシンクした音声を追加することで作ったDeepfake動画のデータセット。ディープフェイクへの対抗策の研究が目的。
2021

AI by Jennifer Tang
OpenAIの言語モデルGPT3を用いた即興劇。ロンドンの劇場での公演。
2021

Turian, J., Shier, J., Tzanetakis, G., McNally, K., & Henry, M. (2021). One Billion Audio Sounds from GPU-enabled Modular Synthesis.
なんと総時間は約126年分!! データセットを生成するためにpytorch上に実装された、GPUに最適化されたモジュラーシンセ torchsynthも合わせて公開。
2021

OpenAI CLIP + Image Generation Models = CLIP art
画像とテキストの関連性の高さを判定するモデルCLIPを用いて、生成される画像を最適化 → テキストの入力にあった画像が生成
2021

The Flemish Scrollers, Dries Depoorter (2021)
顔認識とオブジェクト認識を組み合わせて、議会中に電話をいじっている議員を摘発
2021

Chen, B.-Y., Smith, J. B. L. and Yang, Y.-H. (2020) ‘Neural Loop Combiner: Neural Network Models for Assessing the Compatibility of Loops’.
現代の音楽制作過程で重要なループの組み合わせ。たくさんあるループ間の相性を判定して、適切なループの組み合わせをレコメンドする仕組み。
2020

Algorithms of Late-Capitalism (2020-)
AIや機械学習が社会で用いられる場合、時にそれが市民を搾取するかたちで使われることがある。そうしたAIの利用の実例を取り上げてカタログ化するDigital Activism。
2020

Paint with Music - Google Magenta
Google Magentaチームの最新のプロジェクト。2020年に発表した DDSP: Differentiable Digital Signal Processing を使って、絵筆のストロークを楽器音に変えている。筆で描くように音を奏でることができる。
2021

Knotts, S., & Collins, N. (2020). A survey on the uptake of Music AI Software. Proceedings of the International Conference on New Interfaces for Musical Expression, 594–600.
AI音楽ツールに対する意識調査を117名に対してオンラインで行った。結論からいうと... AIツールはほとんど使われていない。AIツールに対してポジティブな人も、現状ではなく未来の可能性にひかれている。
2020

Alexander Schubert - Convergence (2020)
GANやAutoEncoderが使われている。2021年のアルスエレクトロニカ Digital Musics & Sound Art 部門のゴールデンニカ(最優秀賞)。
2020

-
バイアスやプライバシーへの配慮から、人を対象にしたデータセットを集めることが難しくなっている。そこでGANなどを使って、適切なデータセットを人工的に作り出す仕組みを提供するスタートアップが登場した。
2021

Emma Strubell, Ananya Ganesh, Andrew McCallum (2019)
GPT-2などの言語モデルについて、その精度ではなく、学習時に消費している電力及び、二酸化炭素の放出量についてまとめた。この研究の試算では、例えばTransformer の学習に、一般的な自動車のライフサイクルの約5台分、アメリカ人約17人の一年分に相当するカーボンフットプリントがあることがわかった。
2019

Vigliensoni, G., Mccallum, L., & Fiebrink, R. (2020). Creating Latent Spaces for Modern Music Genre Rhythms Using Minimal Training Data. Proc. ICCC 2020.
少量の学習データでも効率的に学習できるように、最近のダンスミュージックの特徴を生かしたアーキテクチャを採用
2020

Chandna, P., Ramires, A., Serra, X., & Gómez, E. (2021). LoopNet: Musical Loop Synthesis Conditioned On Intuitive Musical Parameters.
音源分離のモデルで提案されたWave-U-Netのアーキテクチャを用いて、ドラムループをまるごと生成する仕組み
2021

Ramires, A., Chandna, P., Favory, X., Gómez, E., & Serra, X. (2019). Neural Percussive Synthesis Parameterised by High-Level Timbral Features. ICASSP, IEEE International Conference on Acoustics, Speech and Signal Processing - Proceedings, 2020-May, 786–790. Retrieved from http://arxiv.org/abs/1911.11853
2019

Zhu, Z., Huang, G., Deng, J., Ye, Y., Huang, J., Chen, X., … Zhou, J. (2021). WebFace260M: A Benchmark Unveiling the Power of Million-Scale Deep Face Recognition
400万人の人の2億6000万枚の顔写真のデータセットと、ゴミデータを取り除いた200万人/4200万枚の顔写真のデータセットの二種類を提供
2021

Ozakar, R., Gazanfer, R. E., & Sinan Hanay, Y. (2020, November 25). Measuring happiness around the World through artificial intelligence
世界8都市の街中で撮られた動画から顔を抜き出してCNNを用いた感情推定モデルで解析。都市ごとの有意な差は見当たらないという結果に。
2020
.png&w=1920&q=90)
Tikhonov, A., & Yamshchikov, I. (2021, July 13). Artificial Neural Networks Jamming on the Beat. 37–44. https://doi.org/10.5220/0010461200370044
リズムパターンのデータセットもあわせて公開。
2021

Behzad Haki, & Jorda, S. (2019). A Bassline Generation System Based on Sequence-to-Sequence Learning. Proceedings of the International Conference on New Interfaces for Musical Expression, 204–209.
2019年のNIMEで発表された論文。最新の言語モデル(seq-to-seq model)の知見を利用してドラムトラックの音声ファイルからそれにあったベースラインを生成してくれる。
2019

Engel, J. et al. (2017) ‘Neural Audio Synthesis of Musical Notes with WaveNet Autoencoders’. Available
WaveNetの仕組みを使ったAutoencoderで、楽器の音の時間方向の変化も含めて、潜在空間にマッピング → 潜在ベクトルから楽器の音を合成する。この研究で使った多数の楽器の音を集めたデータセット NSynth を合わせて公開。
2017

Dmitry Bogdanov, et al. 2013. ESSENTIA: an open-source library for sound and music analysis. In Proceedings of the 21st ACM international conference on Multimedia (MM '13). Association for Computing Machinery, New York, NY, USA, 855–858. DOI:https://doi.org/10.1145/2502081.2502229
https://github.com/MTG/essentia
https://github.com/MTG/essentia

António Ramires, Pritish Chandna, Xavier Favory, Emilia Gómez, & Xavier Serra. (2020). Freesound One-Shot Percussive Sounds (Version 1.0) [Data set]. Zenodo. http://doi.org/10.5281/zenodo.3665275
ドラム、パーカションのワンショットを集めたデータセット
2020

Wu, S.-L. and Yang, Y.-H. (2021) ‘MuseMorphose: Full-Song and Fine-Grained Music Style Transfer with Just One Transformer VAE’
長期の時間依存性を学習できるTransformerの利点とコントロール性が高いVAEの利点。この二つを組み合わせたEncoder-Decoderアーキテクチャで、MIDIで表現された音楽のスタイル変換(Style Transfer)を実現。
2021

Hertzmann, A. (2020) ‘Visual indeterminacy in GAN art’, Leonardo. MIT Press Journals, 53(4), pp. 424–428.
GANが生成する画像の「●●ぽいけど、なんか違う...」という「不確定性」に着目し、現代アートの特徴との比較を行った上で、今後のGANアートの将来像を探る。
2020

Madhu, A. and K, S. (2021) ‘EnvGAN: Adversarial Synthesis of Environmental Sounds for Data Augmentation’.
環境音の識別モデルの学習のためのData Augmentation手法の提案
2021

Child, R. et al. (2019) ‘Generating Long Sequences with Sparse Transformers’, arXiv. arXiv. Available at: http://arxiv.org/abs/1904.10509 (Accessed: 29 January 2021).
スパースなTransformerの仕組みで計算量を抑える
2019
.png&w=1920&q=90)
Welleck, S., Kulikov, I., Roller, S., Dinan, E., Cho, K., & Weston, J. (2019). Neural Text Generation with Unlikelihood Training.
Likelihoodを最適化しようとすると頻出する単語が必要以上に頻出する結果に
2019

Gangadharbatla, H. (2021) ‘The Role of AI Attribution Knowledge in the Evaluation of Artwork’, pp. 1–19. doi: 10.1177/0276237421994697.
クラウドソーシングの仕組みを活用して、AIが描いた絵を区別できるか、またAIが描いたという情報が、絵自体の評価にどのくらい影響するのかを調査。
2021

Harvey, Adam. LaPlace, Jules, Exposing.AI
Flickrなどに顔写真をアップロードした場合、特にCreative Commonsのライセンスなどを設定した場合、知らない間に顔認識モデルの学習データとして使われている可能性がある。このサイトは自分の写真が、学習データとして使われていないかチェックできるサイト。
2021

Infinite Bad Guy (IYOIYO, Kyle McDonald)
YouTube上で史上最も多くカバーされた楽曲ビリー・アイリッシュのBad Guy。YouTube上にあがっている曲を解析、分類し、リズムに合わせてスムーズに繋いでいく。無限に続くBad Guyジュークボックス。
2020

Hutchings, P. (2017). Talking Drums: Generating drum grooves with neural networks.
ドラムのキックの位置を入力すると、リズムパターン全体を生成するモデル。言語モデルのseq-to-seqモデルの考え方を利用。
2017

UAV-Human: A Large Benchmark for Human Behavior Understanding with Unmanned Aerial Vehicles
ドローンから撮影した人の行動の動画のデータセット。ドローンを使った監視に直結する恐れ??
2021

random_faces
ライブラリをインストールしてたった二行で、存在しない人の顔画像が生成できるPythonライブラリ
2021

"AAI" by Mouse on Mars
ベテラン電子音楽家、あのMouse on Marsが、AIを用いたアルバムを公開!! テキストを入力した声を合成するシステムを構築。生成した声を用いて楽曲を構成した。
2021

ArtEmis: Affective Language for Visual Art
8万枚の絵画にクラウドソーシングで44万の言語情報を付加。
2021

Verma, P., Basica, C. and Kivelson, P. D. (2020) ‘Translating Paintings Into Music Using Neural Networks’.
音楽とアルバムカバーの関係を学習したモデルをベースに、絵画と音楽を相互に変換するパフォーマンス
2020

Francesco Ganis, Erik Frej Knudesn, Søren V. K. Lyster, Robin Otterbein, David Südholt, Cumhur Erkut (2021)
Google MagentaのDDSPをリアルタイムに動かせるプラグイン
2021

Vaswani, A., Shazeer, N., Parmar, N., Uszkoreit, J., Jones, L., Gomez, A. N., Kaiser, Ł., & Polosukhin, I. (2017). Attention is all you need. Advances in Neural Information Processing Systems, 2017-Decem, 5999–6009.
現在、GPT-3から音楽生成、画像の生成まで、多様な領域で中心的な仕組みとなっているTransformerを導入した論文。時系列データの学習に一般的に用いられてきたRNNなどの複雑なネットワークを排して、比較的シンプルなAttentionだけで学習できることを示した。
2017

Hertzmann, A. (2020) ‘Visual indeterminacy in GAN art’, Leonardo. MIT Press Journals, 53(4), pp. 424–428. doi: 10.1162/LEON_a_01930.
グラニュラーシンセシスのGrain(音の粒)をVAEを使って生成しようという試み。Grainの空間の中での軌跡についても合わせて学習。
2020

CinemaNet by Anton Marini(vade), Rahul Somani
普通の画像認識モデルのようなオブジェクトの識別に加えて、カメラのアングルやフォーカスの当て方(ソフトフォーカス...)、撮影された時間帯(夕方、朝焼け)、場所などをタグ付け

Mor, Noam, et al. "A universal music translation network." arXiv preprint arXiv:1805.07848 (2018).
2018

Lee, Hsin-Ying, et al. "Dancing to music." arXiv preprint arXiv:1911.02001 (2019)
2019

Han-Hung Lee, Da-Gin Wu, and Hwann-Tzong Chen, "Stylizing Audio Reactive Visuals", NeurlPS2019, (2019)
2019

Piano Genie
2018

Yu, Yi, Abhishek Srivastava, and Simon Canales. "Conditional lstm-gan for melody generation from lyrics." ACM Transactions on Multimedia Computing, Communications, and Applications (TOMM) 17.1 (2021): 1-20.

Tatsuki Koga, at el., "Human and GAN collaboration to create haute couture dress", 33rd Conference on Neural Information Processing Systems, (2019)
2019

Loh, Bryan, and Tom White. "Spacesheets: Interactive latent space exploration through a spreadsheet interface." (2018).
2018

谷口忠大, "記号創発問題: 記号創発ロボティクスによる記号接地問題の本質的解決に向けて (< 特集> 認知科学と記号創発ロボティクス: 実世界情報に基づく知覚的シンボルシステムの構成論的理解に向けて)", 人工知能 Vol.31.1, pp74-81, (2016).
2016

様々なメディアのフレームを補間する – Depth-Aware Video Frame Interpolation
2020

Huang, Sicong, et al. "Timbretron: A wavenet (cyclegan (cqt (audio))) pipeline for musical timbre transfer." arXiv preprint arXiv:1811.09620 (2018).
2018

SPLEETER: A FAST AND STATE-OF-THE ART MUSIC SOURCE SEPARATION TOOL WITH PRE-TRAINED MODELS
CDなどのミックスされた音源からボーカル、ピアノ、ベース、ドラムのようにそれぞれの楽器(トラック)の音を抽出できるツール
2019

2.5D Visual Sound
2019

Dan Hendrycks, Kevin Zhao, Steven Basart, Jacob Stein- hardt, and Dawn Song. Natural adversarial examples. arXiv preprint arXiv:1907.07174, 2019.
2019
Artist in the Cloud: Towards an Autonomous Artist
自律型人工アーティストの制作を試みるオープンプレジェクト
2019

Audio-Visual Scene Analysis with Self-Supervised Multisensory Features
2018

Lehman, Joel, et al. "The surprising creativity of digital evolution: A collection of anecdotes from the evolutionary computation and artificial life research communities." Artificial life 26.2 (2020): 274-306.
2020

Synthesizing Audio with Generative Adversarial Networks
2018

This CAPTCHA Makes Sure You're A Robot. Yes, You Read That Right

Visual to Sound: Generating Natural Sound for Videos in the Wild
2018

Improvised Comedy as a Turing Test
RNNベースのseq2seq会話エンジンを持ったロボットを交えた即興の漫才. 二人のコメディアン兼研究者とロボットのショー
2017

Neural 3D Mesh Renderer
2017

Deep Learning Techniques for Music Generation – A Survey
2017

Maison book girl 「cotoeri」
2017

The Electronic Curator
2017

Sherry, AI-Powered Horror Stories
2017

Automatically identifying wild animals in camera-trap images with deep learning
アフリカの野生動物の観測にDeep Learningを利用 – Automatically identifying wild animals in camera-trap images with deep learning
2017

AVA: A Video Dataset of Spatio-temporally Localized Atomic Visual Actions
2017

Delusions
2017

A Dictionary of Consciousness
2017
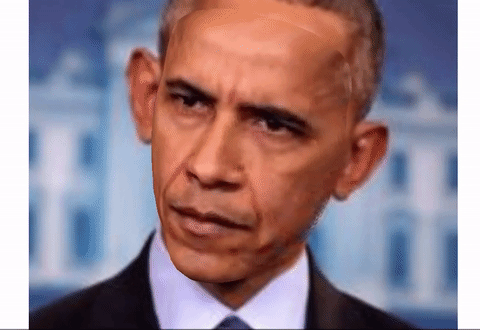
Large Pose 3D Face Reconstruction from a Single Image via Direct Volumetric CNN Regression
2017

Deep Learningを活用した都市の”形態学”
Deep Learningを活用した都市の”形態学”
2017

A Machine Learning Approach for Evaluating Creative Artifacts
「人間の創造性をどのように評価、定量化するか」という大きな問題に取り組んでいる
2017

GrooveNet: Real-Time Music-Driven Dance Movement Generation using Artificial Neural Networks
音楽の特徴に基づいたダンスの動きのリアルタイム生成
2017

グラフィックデザインにおける各要素の重要性を可視化 – Learning Visual Importance for Graphic Designs and Data Visualizations
グラフィックデザインにおける各要素の重要性を可視化
2017

衛星写真から住民の所得を推定 – Penny, an AI to predict wealth from space
衛星写真から住民の所得を推定
2017

Penny, an AI to predict wealth from space
2017

Learning Cross-modal Embeddings for Cooking Recipes and Food Images
料理の写真 ↔︎ 材料とレシピ
2017

The iNaturalist Challenge 2017 Dataset
2017

Yang, Li-Chia, Szu-Yu Chou, and Yi-Hsuan Yang. "Midinet: A convolutional generative adversarial network for symbolic-domain music generation." arXiv preprint arXiv:1703.10847 (2017).
GANで音楽生成
2017

Performance RNN: Generating Music with Expressive Timing and Dynamics
2017

CAN: Creative Adversarial Networks Generating “Art” by Learning About Styles and Deviating from Style Norms
2017

Interactive 3D Modeling with a Generative Adversarial Network
2017

Chandrasekaran, Arjun, Devi Parikh, and Mohit Bansal. "Punny captions: Witty wordplay in image descriptions." arXiv preprint arXiv:1704.08224 (2017).
2017

Musical Novelty Search – Evolutionary Algorithms + Ableton Live
2017

2017

Deep Cross-Modal Audio-Visual Generation
2017

See, Hear, and Read: Deep Aligned Representations
2017

Mapillary Vistas Dataset
2017

適切なフォントの組み合わせを生成 – Fontjoy
適切なフォントの組み合わせを生成 – Fontjoy
2017

Generating Videos with Scene Dynamics
2017

Forecasting Human Dynamics from Static Images
2017

AIを言葉でナビして学習 – Beating Atari with Natural Language Guided Reinforcement Learning
AIを言葉でナビして学習 – Beating Atari with Natural Language Guided Reinforcement Learning
2017

Beyond Face Rotation: Global and Local Perception GAN for Photorealistic and Identity Preserving Frontal View Synthesis
2017

絵を「描く」プロセスの模倣 – A Neural Representation of Sketch Drawings
絵を「描く」プロセスの模倣 – A Neural Representation of Sketch Drawings
2017

目線をあやつる – Gaze Warping
目線をあやつる – Gaze Warping
2017

The Infinite Drum Machine : Thousands of everyday sounds, organized using machine learning
2017

Seeing Invisible Poses: Estimating 3D Body Pose from Egocentric Video
2017

Photo Aesthetics Ranking Network with Attributes and Content Adaptation
2017

ピクセルではなく、ベクターで画像を生成する。
ピクセルではなく、ベクターで画像を生成する。
2016

Transfer Learning - Machine Learning's Next Frontier
Transfer Learning - Machine Learning's Next Frontier
2017

AutoHair: Fully Automatic Hair Modeling from A Single Image
2017

Unpaired Image-to-Image Translation using Cycle-Consistent Adversarial Networks
2017

Using Deep Learning and Google Street View to Estimate the Demographic Makeup of the US
2017

Colormind
2017

AIで表現するとは?壮大なデジタルの歴史から紐解く、その可能性について
2017

Changing Fashion Cultures
2017

Abe, Kaori, et al., "Changing fashion cultures." arXiv preprint arXiv:1703.07920, (2017)
ファッションの地理的および時系列的なトレンドをスナップ写真から解析するプロジェクト.
2017

DONAHUE, Chris; LIPTON, Zachary C.; MCAULEY, Julian, "Dance dance convolution. In: International conference on machine learning", PMLR, pp. 1039-1048, (2017)
CNNとLSTMでダンスダンスレボリューションのステップ譜
2017

Schifanella, Rossano, Miriam Redi, and Luca Maria Aiello, "An image is worth more than a thousand favorites: Surfacing the hidden beauty of flickr pictures.", Ninth International AAAI Conference on Web and Social Media, (2015)
2015

AudioSet
2017

Collection of over 200,000 short jokes for humour research
2017

Using human brain activity to guide machine learning
2017

Deep Photo Style Transfer
2017

Learning to Generate Posters of Scientific Papers
2017

HE, Kaiming, et al., "Mask r-cnn", Proceedings of the IEEE international conference on computer vision, pp. 2961-2969, (2017)
Faster-RCNNの拡張. ひとつのモデルで最小限の変更で物体検出、輪郭検出、人の姿勢の検出を高い精度で行う.
2017

DeepWarp: Photorealistic Image Resynthesis for Gaze Manipulation
2017

DeepDreamを用いたのドローイングツール- DreamCanvas
DeepDreamを用いたのドローイングツール- DreamCanvas
2017

GANを応用したSANによるSaliency Map(顕著性マップ)の生成 – Supervised Adversarial Networks for Image Saliency Detection –
GANを応用したSANによるSaliency Map(顕著性マップ)の生成
2017

機械学習を用いたドローイングツール – AutoDraw
機械学習を用いたドローイングツール – AutoDraw
2017

Domenech, Arnau Pons, and Hartmut Ruhl. "An implicit ODE-based numerical solver for the simulation of the Heisenberg-Euler equations in 3+ 1 dimensions." arXiv preprint arXiv:1607.00253 (2016).
2016

magenta session
2017

Convolutional Recurrent Neural Networks for Bird Audio Detection
2017

Face-to-BMI: Using Computer Vision to Infer Body Mass Index on Social Media
2017

RobotArt – the Robot Art competition
the Robot Art competition
2017

ファッション写真のデータセット – Large-scale Fashion (DeepFashion) Database
2016

日々のニュース写真から似通った作品を検索
日々のニュース写真から似通った作品を検索
2017

LIU, Ziwei, et al., "Video frame synthesis using deep voxel flow", Proceedings of the IEEE International Conference on Computer Vision, pp. 4463-4471, (2017)
2017

Deep Clustering and Conventional Networks for Music Separation: Stronger Together
2016

YANG, Shuai, et al. "Awesome typography: Statistics-based text effects transfer", Proceedings of the IEEE Conference on Computer Vision and Pattern Recognition, pp.7464-7473, (2017)
2017

OPUS – the open parallel corpus
2017

food2vec – Augmented cooking with machine intelligence
2017

food2vec – Augmented cooking with machine intelligence
2016

Learning to Draw: Generating Icons and Hieroglyphs
2017

How many animals can one find in a random image?
2017

AENet: Learning Deep Audio Features for Video Analysis
2017

T-SNE MAP – Google Arts and Culture Experiments
2016

Abadi, Martín, and David G. Andersen. "Learning to protect communications with adversarial neural cryptography." arXiv preprint arXiv:1610.06918 (2016)
2016

The Automation of Creativity: How man & AI will work together to improve the ad industry
2017

ドキュメンタリー – The Automation of Creativity: How man & AI will work together to improve the ad industry
2016

Imaginary landscapes using pix2pix
2016

2016

The Lakh MIDI Dataset v0.1
2016

Hadjeres, Gaëtan, François Pachet, and Frank Nielsen, "Deepbach: a steerable model for bach chorales generation.", International Conference on Machine Learning. PMLR, (2017)
2017

Unsupervised Learning of 3D Structure from Images
2016

Aytar, Yusuf, Carl Vondrick, and Antonio Torralba, "Soundnet: Learning sound representations from unlabeled video.", Advances in neural information processing systems 29, pp892-900 (2016)
2016

Malik, Iman, and Carl Henrik Ek. "Neural translation of musical style." arXiv preprint arXiv:1708.03535 (2017).
2017

Portraits of Imaginary People
2021
2018